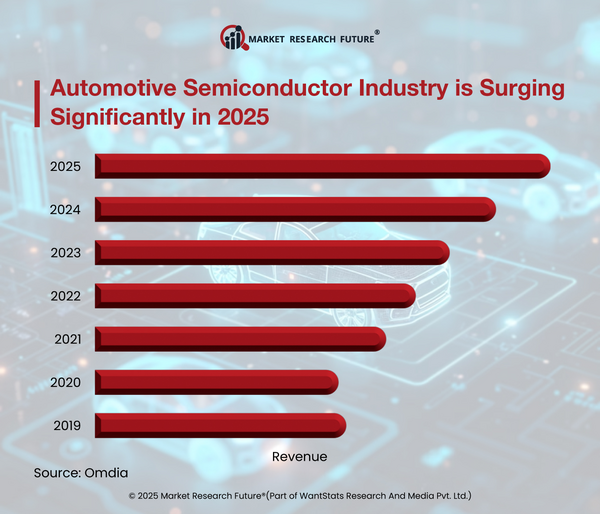
Deep Learning Estimates Watershed Subsurface Permeability using Stream Discharge
By Aarti Dhapte , 21 November, 2022
Scientists from Pacific Northwest National Laboratory, Oak Ridge National Laboratory, and Los Alamos National Laboratory trained deep neural networks (DNNs) to estimate subsurface permeability from stream discharge hydrographs in a study published in Frontiers in Earth Science.
In watershed models, subsurface permeability is a critical parameter that determines the contribution of subsurface flow to stream flows. It is difficult and expensive to directly measure permeability at the spatial extent and resolution required by the watershed models. Researchers often use inverse modeling to evaluate permeability. Compared to groundwater monitoring data, the wide availability of stream surface flow data provides a novel data source for integrated surface and subsurface hydrologic models to infer soil and geologic features.
First, they trained the DNNs to map the correlations between soil and geologic layer permeabilities and simulated stream discharge from the examined watershed's integrated surface-subsurface hydrologic model. The DNNs outperformed the classic inverse modeling method in terms of permeability estimates. Using observed stream discharge from the research site, the DNNs assessed the permeability of a natural watershed (Rock Creek Catchment in the headwaters of the Colorado River). The watershed model accurately predicted the stream flows, with permeability calculated by DNNs. This study highlights the importance of upcoming deep learning methods for assisting with integrated watershed modeling by enhancing parameter estimation, which will eventually reduce uncertainty in predictive watershed models.
The permeability of subsurface rocks and soils measures how well liquids flow through them. It is essential in determining subsurface flow and transport mechanisms in watersheds. However, measuring permeability directly at the size and precision required by watershed models is challenging and expensive. Stream flow monitoring data, on the other hand, is publicly available. The connections between permeability and stream flow open a new avenue for evaluating subsurface permeability. Deep learning, a sort of artificial intelligence, was used in this study. Deep understanding assesses a watershed's subsurface permeability from stream discharge data more precisely than standard methods. This enhancement will aid in calibrating watershed models and minimize the uncertainty in stream discharge prediction.
Traditional Machine Learning and Deep Learning Performance Comparison
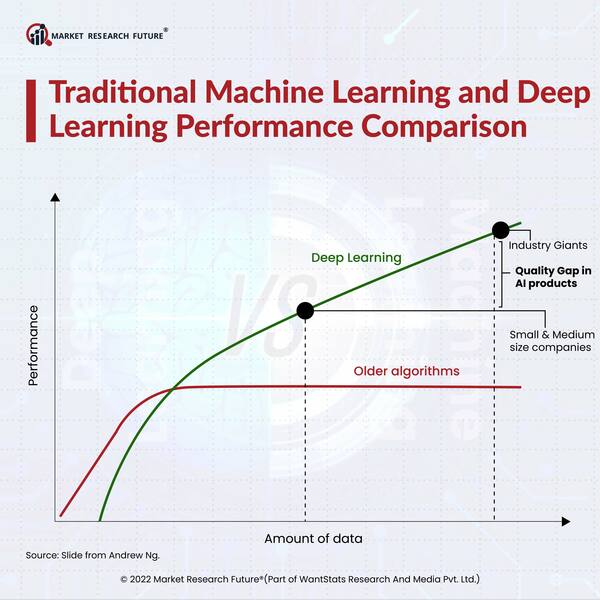
Latest News
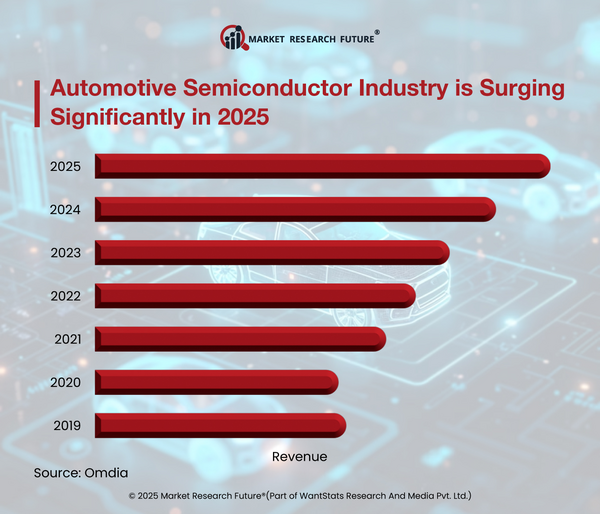
The automotive industry will benefit significantly from strategic partnerships in artificial intelligence (AI) and its use in cars. AI integration into automobiles is projected to improve considerably in the year 2025 due to the formation of various…
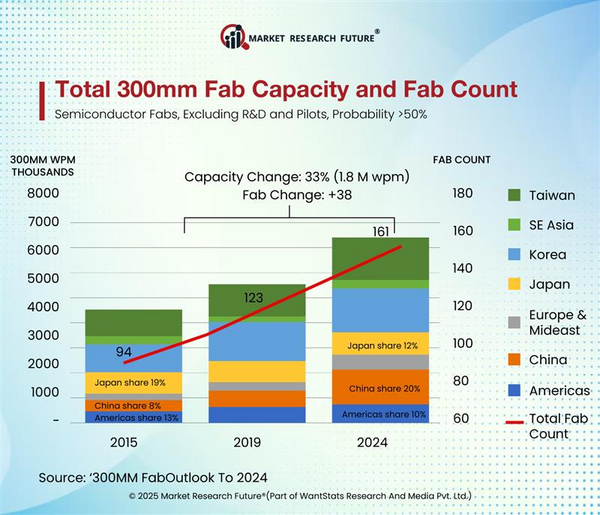
In 2025, the global semiconductor industry is already grappling with new and complex challenges emanating from the trade wars and policy changes between the United States and China. The semiconductor industry, an essential backbone of technology…
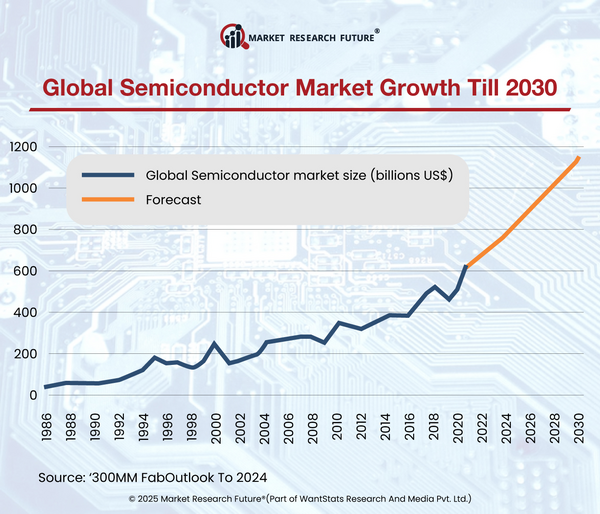
The rapid use of generative artificial intelligence (AI) in 2025 has profoundly affected the semiconductor sector, resulting in unprecedented growth for advanced chip technologies. According to estimates made by the Capgemini Research Institute, this…
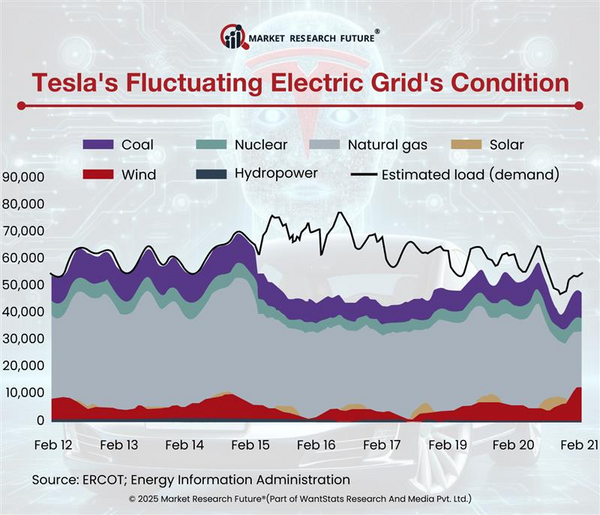
Texas will face an emerging challenge in 2025 as the electricity grid experiences unprecedented strain from the rapid expansion of artificial intelligence (AI) data centers. Power usage has increased due to technology firms' indiscriminate placement…
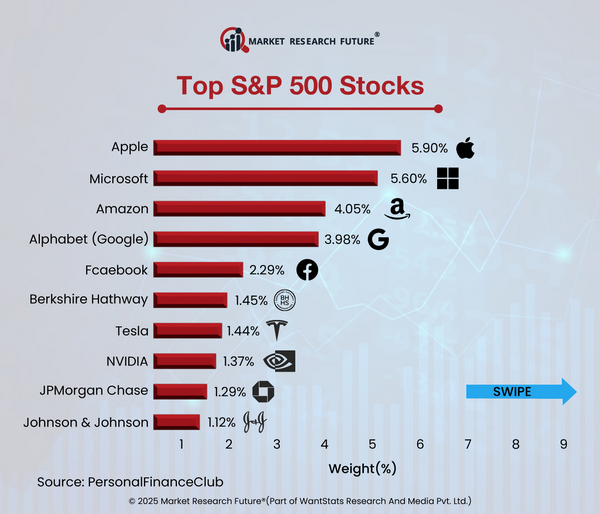
With the "Magnificent Seven”- Apple, Microsoft, Amazon, Alphabet, Meta, Nvidia, and Tesla leading the fall, the US stock market saw a significant dip in early 2025. Usually seen as market drivers, these IT behemoths found themselves in the correction…

Team Lead - Research
Latest News
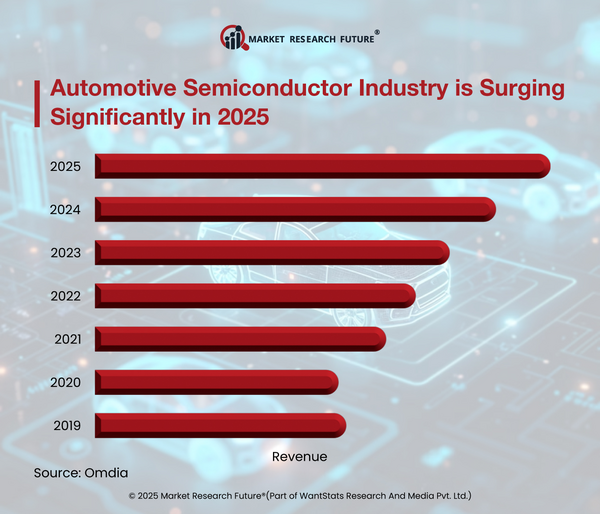
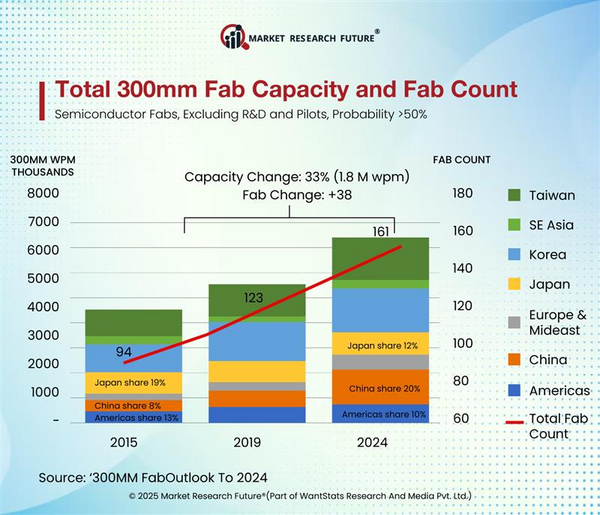
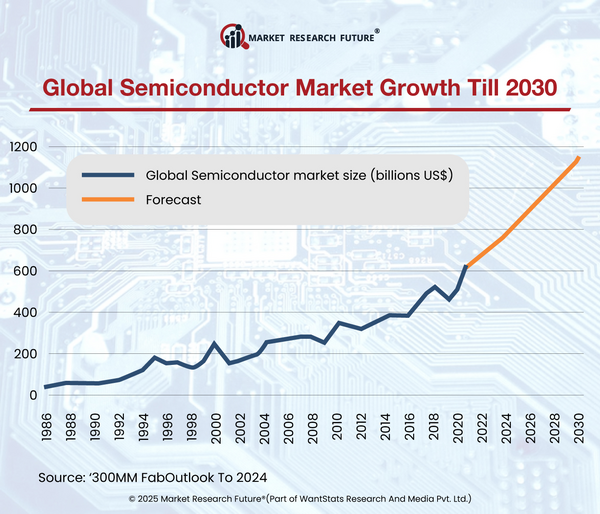
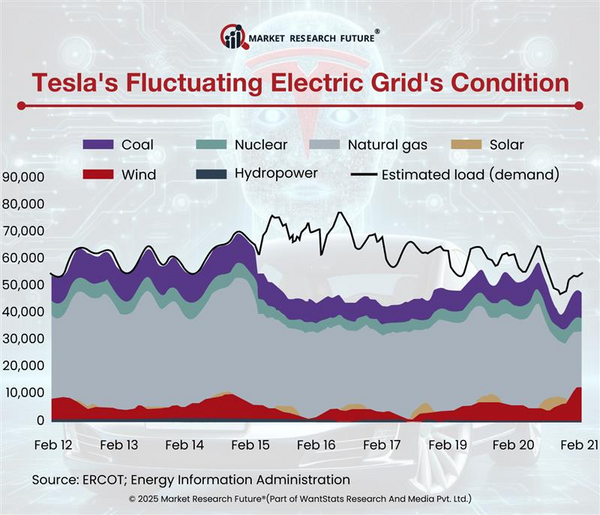
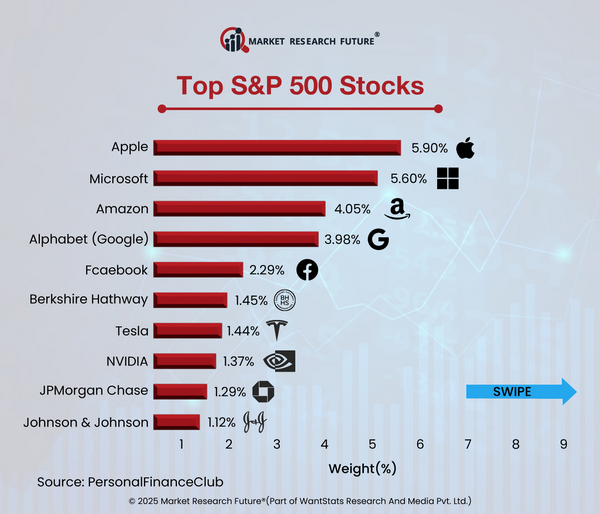